Measuring Research ROI: The One Metric to Rule Them All (Just Kidding—It’s Chaos)
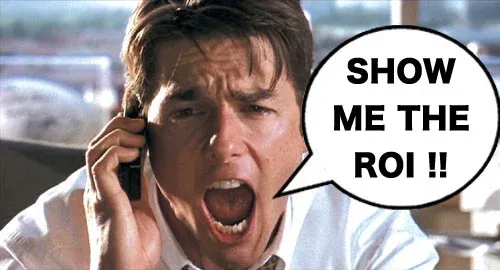
"What's the ROI of Research?" he asked, adjusting his KPI monocle while sipping from a mug emblazoned with "DISRUPT OR DIE."
The ROI question. The corporate equivalent of asking a chef to quantify the exact financial return of salt. Sure, we could serve unseasoned food, but have you tasted it? No? Well, neither have our executive stakeholders tasted the unsalted soup of launching products without research. But they're quite certain it would be fine. Probably delicious, even! After all, they like it.
Welcome to the glorious theater of attempting to justify understanding humans before building things for them. Pour yourself a metrics-driven cocktail and join me on this journey through the most circular logic puzzle in business today—a journey where we will explore the bizarre rituals of corporate ROI calculation, the strange mythology of measurement, and the peculiar psychology that leads intelligent people to question the value of understanding their users.
The Corporate Time Machine That Never Was
Imagine, if you will, a parallel universe where companies can simultaneously launch two identical products—one informed by research and one created purely from executive hunches and whatever the CEO saw on TikTok last week. In this magical realm, we could measure the precise impact of research on user satisfaction, conversion rates, and lifetime value.
Sadly, we don't live in that universe. We live in this one, where product leaders earnestly ask, "But how do we know the research mattered?" shortly after launching a product that didn't crash and burn specifically because we tested it with users beforehand.
It's rather like asking what the ROI was on that time you decided not to drive blindfolded on the highway. The return? You arrived alive! But since the counterfactual scenario involves a fiery wreck that never happened, it's hard to put a dollar value on it.
"We need to measure everything," declares your analytics team, who cannot tell you how many users abandoned your product last week due to confusion but can produce seventeen dashboards showing how many clicked a button that doesn't actually do anything. These are the same people who present quarterly reports with more line charts than a cardiac monitor, but somehow never include the chart labeled "Features We Built That No One Uses Because We Didn't Check If Anyone Wanted Them."
I once had an analytics director—let's call him Graph Gary—who insisted we couldn't justify our research program without "hard numbers." This was the same person who couldn't explain why our conversion rate dropped 30% after we implemented his "data-driven" redesign (a redesign that, coincidentally, ignored all the research insights showing users found it confusing). When I suggested we might want to understand why metrics were dropping by—crazy idea incoming—talking to actual humans, he scoffed and said, "The numbers will tell us everything."
Three months and four emergency research sprints later, we discovered users were abandoning the site because they couldn't find the checkout button that Gary had helpfully hidden behind a hamburger menu to "clean up the UI." The ROI of that discovery? Merely saving the entire revenue stream of the company. But naturally, we had to justify our research budget again the following quarter.
The ROI Contortionist Act
When pressed for the hallowed ROI number, researchers around the world perform the same ritual: we stretch, we reach, we bend ourselves into impossible positions attempting to draw direct lines between "we talked to users" and "money appeared."
"Well," we begin, already knowing what's coming, "our usability testing identified 23 critical issues that would have prevented users from completing the checkout flow."
"But how much money did that make us?" asks the executive, who has never personally attempted to use the company's product and whose laptop still has the factory default screensaver because IT set it up for them.
"By fixing those issues, we improved conversion by 12%."
"But how do we know it was the research that caused that?"
And there it is—the question that assumes research is a magical revenue lever rather than the thing that told us which levers to pull in the first place.
The truth is, research doesn't generate revenue directly. It's the corporate equivalent of a map. Maps don't get you to your destination—they just dramatically increase your chances of not ending up in a ditch. But try explaining that to someone who believes the only valuable business activities are those with immediately quantifiable returns.
In my darker moments, I imagine alternative scenarios:
Surgeon: "I'd like to do an MRI before operating."
Hospital Administrator: "What's the ROI of knowing where the tumor is?"
Pilot: "I'd like to check the weather before takeoff."
Airline CEO: "But can you quantify the exact financial return of not flying into a hurricane?"
Civil Engineer: "We should test the soil before building this skyscraper." Developer: "Do we really need to spend money understanding the ground? Can't we just start pouring concrete and see what happens?"
Yet somehow, in product development, flying blind is considered a viable strategy, and researching beforehand is a luxury that must be justified with elaborate ROI theater.
The Mythical "Research ROI Calculator"
If I had a dollar for every time someone asked for a "simple formula" to calculate research ROI, I could fund my own research practice and never have this conversation again.
The fantasy goes something like this:
Research ROI = (Money Made After Research - Money Made Before Research) / Cost of Research
Beautiful in its simplicity. Utterly divorced from reality.
Here's a more accurate formula:
Research Value = (Disasters Avoided × Cost of Each Potential Disaster) + (Good Ideas Pursued × Value of Not Wasting Time) + (Insights That Changed Direction × Impossible to Calculate Factor) - (Insights Ignored × Why Did We Even Bother Coefficient)
But that doesn't fit neatly into a quarterly business review slide, so we continue the charade.
Let's get technical for a moment, shall we? If we were to genuinely attempt to calculate research ROI, we would need:
- A perfect counterfactual (what would have happened without research)
- Perfect attribution (which outcomes were caused specifically by research insights)
- Perfect valuation of prevented disasters (how much it would have cost if we'd built the wrong thing)
None of these are possible. It's like trying to calculate the ROI of your car's brakes by measuring how many accidents you didn't have.
But we're not really operating in a realm of rational calculation. We're operating in a corporate mythos where the appearance of measurement has become more important than the reality of understanding. So we create elaborate formulas that give the illusion of precision while masking the fundamental absurdity of the exercise.
The Sacred "Saving Engineering Time" Argument
When all else fails, there's always the engineering time argument—the one calculation that makes even the most research-resistant executive pause.
"Our concept testing revealed that users have zero interest in the blockchain-enabled virtual pet feature. We estimate this saved approximately 1,200 engineering hours."
And suddenly, research has value! Not because it prevented a terrible user experience, not because it helped us understand human needs, but because it saved engineering resources—the only corporate resource that apparently has universally agreed-upon value.
The fact that this argument works reveals the absurdity of the ROI question itself. We value avoiding wasted engineering time because it has a clear cost. But we struggle to value creating good user experiences because the benefit is diffuse, long-term, and—how do I put this delicately—actually related to how humans experience your product rather than how much it cost to build.
I call this the "Engineering Hour Exchange Rate." In most companies, one hour of engineering time is roughly equivalent to:
- 5 hours of design time
- 10 hours of research time
- 100 hours of user frustration
- ∞ hours of customer support dealing with confused users
This exchange rate tells you everything you need to know about corporate priorities. Engineering is the gold standard against which all other activities are measured. Research is the corporate equivalent of the Estonian Kroon—technically currency, but nobody's quite sure of the exchange rate.
To leverage this reality, I've started translating all research outcomes into "engineering hours saved." User interviews revealed customers want a simpler workflow? That's 400 engineering hours saved on building features they wouldn't use. Usability testing showed navigation issues? That's 250 engineering hours saved on building the wrong solution.
Is this intellectually honest? Not entirely. But in the land of the ROI blind, the person with engineering hour calculations is king.
Let's put some real numbers to this. At a typical tech company:
- Average fully-loaded cost of an engineer: $200,000/year
- Hours worked per year: 2,080
- Cost per engineering hour: ~$96
So when research prevents a team of five engineers from spending three months building something users don't want:
5 engineers × 3 months × 160 hours/month × $96/hour = $230,400 saved
This calculation doesn't account for opportunity cost (what those engineers could have built instead), future maintenance burden of unwanted features, or user goodwill preserved. But it's a number that fits in a spreadsheet, and in the corporate world, that's sometimes all that matters.
Case Study: The Great "Users Hate This" Revelation
Let me tell you about Project Avalanche—so named because it ultimately buried its stakeholders in user complaints.
The executive vision was clear: users would love a complete redesign that removed all the "unnecessary" steps in our core workflow. When research suggested testing this assumption, we were told there wasn't time, budget, or need since "it's obviously better."
Three months and $1.2 million in development costs later, the new streamlined experience launched. Within 24 hours, support tickets increased by 340%. User forums erupted with confusion. The CEO received personal emails from our largest customers asking if we'd been hacked.
Two weeks later, we reverted to the previous design after emergency backlog reprioritization, all-hands engineering sprints, and a very tense board meeting where the CTO developed an eye twitch that medical professionals later confirmed was triggered by the phrase "but we tested it internally."
The post-mortem question wasn't "What was the ROI of the research we didn't do?" It was "How did this happen?" As if it were some unforeseen natural disaster rather than the entirely predictable outcome of not checking whether your assumptions matched reality.
The ROI of the research we didn't do? Negative $1.2 million, plus incalculable brand damage and a product team with collective PTSD. The VP of Product started referring to the incident as "The Unpleasantness" and would change the subject whenever it came up, like a Victorian gentleman avoiding mention of a family scandal.
But that's not the kind of ROI calculation that makes it into planning spreadsheets. It's certainly not the kind that gets presented at the annual shareholder meeting, where executives prefer to focus on "strategic pivots" rather than "completely avoidable disasters that occurred because we didn't spend $50,000 on research."
If we do the math:
Cost of research not done: $50,000
Cost of disaster: $1,200,000
ROI of researched avoided: -2,300%
Now, I'm not a financial expert, but negative two thousand percent seems like a bad investment strategy. Yet companies make this same calculation error repeatedly, because the cost of research is visible and immediate, while the cost of ignorance is hypothetical until suddenly, catastrophically, it isn't.
The Three Horsemen of the Research Apocalypse
When it comes to denying the value of research, executives typically rely on three arguments, each more absurd than the last:
1. "We already know what users want." This is usually proclaimed by someone who hasn't spoken directly to a user since 2017 and whose understanding of "user needs" is based entirely on what customers complain about after you've already built the wrong thing.
This is the "Omniscience Fallacy," the belief that because you use products, you understand all users of products. It's like assuming you understand the needs of all restaurant patrons because you occasionally eat food.
I once worked with a Chief Product Officer who dismissed research findings with, "That can't be right. My wife wouldn't use it that way." His wife, apparently, was the secret avatar for our entire user base of 3.4 million people across 43 countries. Who knew?
2. "We can't afford to slow down." This perspective values speed over direction, as if driving faster while lost is somehow an efficiency improvement. "We need to move quickly!" they declare, racing confidently toward the wrong destination.
This is the "Velocity Vortex"—the organizational black hole that sucks in resources and spits out features, regardless of whether those features solve actual problems. In the Velocity Vortex, the only metric that matters is speed of delivery. The fact that you're delivering things no one wants is irrelevant as long as you're delivering them quickly.
As one engineering director put it to me: "We don't have time to figure out if we're building the right thing. We need to ship this feature by Q3!" The fact that the feature would need to be redesigned in Q4 after users rejected it was apparently a future problem for future people.
3. "The data will tell us everything." Ah yes, the data. Those beautiful quantitative breadcrumbs that tell you what happened but never why. "The data shows users aren't using this feature," says the analyst, unable to explain whether it's because users hate it, can't find it, don't understand it, or were abducted by aliens before completing the flow.
This is the "Quantitative Quicksand"—the belief that if you collect enough metrics, understanding will spontaneously emerge like a phoenix from a pile of pivot tables. In Quantitative Quicksand, a dashboard with enough charts will somehow explain human motivation, even though it can't distinguish between "users don't want this" and "users don't know this exists."
I've sat through meetings where the analytics team proudly announced that "engagement with Feature X is below expectations" but couldn't offer any insight into why. When research suggested talking to users to find out, we were told there wasn't budget for that—but there was apparently plenty of budget for three more analysts to create additional charts confirming that yes, engagement was indeed still below expectations.
Combined, these three horsemen create the perfect storm of product development dysfunction—building the wrong things quickly and then being confused when metrics don't improve.
It's the corporate version of repeatedly hitting yourself in the face with a hammer, collecting detailed metrics on the resulting pain, and then wondering why your headache isn't improving. Perhaps—radical thought incoming—we could stop hitting ourselves with the hammer? Or at least check if there's a better tool for the job?
Alternative ROI: The Return on Ignorance
Perhaps instead of focusing on the Return on Investment for research, we should calculate the Return on Ignorance—the cost of deliberately choosing not to understand your users before making product decisions.
The ROI of ignorance includes:
- The engineering cost of building features nobody uses
- The opportunity cost of not building what users actually need
- The support cost of confused and frustrated users
- The marketing cost of trying to convince users they want what you've built
- The executive time spent in meetings wondering why adoption is low
Unlike the ROI of research, these costs are startlingly concrete. We can measure exactly how much we spent building the feature that has a 0.002% usage rate. We can count the support tickets. We can track the churn.
Let's put some real numbers to this:
Cost of Ignorance = (Engineering Cost of Unused Features) + (Opportunity Cost of Missed Solutions) + (Support Cost of User Confusion) + (Marketing Cost of Persuading Users) + (Executive Time Spent on Postmortems)
For a typical mid-sized product company:
- Engineering cost of unused features: $2M/year (20% of features built are rarely used)
- Opportunity cost of missed solutions: $5M/year (conservative estimate of revenue from unmet user needs)
- Support cost of user confusion: $1M/year (additional tickets, longer resolution times)
- Marketing cost of persuading users: $500K/year (campaigns promoting features users didn't ask for)
- Executive time spent on postmortems: $250K/year (endless meetings about why things aren't working)
Total Cost of Ignorance: $8.75M/year
Compared to a typical research budget of $500K-$1M, the ROI of not being ignorant about your users is approximately 775-1650%.
But acknowledging these costs would require admitting that perhaps—just perhaps—talking to users before building things might be valuable regardless of whether that value fits into a tidy formula.
And admitting that would mean acknowledging that the emperor's new product strategy might be missing some clothing. It's far easier to keep demanding ROI calculations for research while never calculating the cost of flying blind.
The Research Cycle of Grief
Every researcher knows the cycle all too well:
- Denial: "We don't need research for this one—it's obvious what users want." This phase is characterized by confident statements about user needs, usually made by people who haven't spoken to a user in years. The hallmark of this phase is the phrase "I would definitely use it this way," as if personal preference were a reliable proxy for user behavior.
- Launch: The product or feature is released into the wild with much fanfare. Press releases declare it "revolutionary" and "user-centered" despite no users having been centered in its development. This phase often includes a launch party where executives congratulate each other on their visionary leadership.
- Confusion: Users don't react as expected. Metrics don't move. Support is overwhelmed with questions like "How do I find the thing that used to be here?" and "Why did you change this?" This phase is marked by emergency meetings where people stare at dashboards as if they might eventually reveal the secret of human motivation.
- Bargaining: "Maybe we just need better onboarding? A tutorial? A complete redesign?" This is when the blame-shifting begins. It's not that we built the wrong thing—it's that users don't understand how brilliant it is! If only we explained it better with more tooltips, pop-ups, and product tours that no one reads!
- Research: Finally, someone suggests talking to users to understand what went wrong. This suggestion is met with reluctance but eventually accepted as a last resort. "Fine, we'll do some research, but make it quick and cheap."
- Insight: "Oh, users actually needed something completely different than what we built." The research reveals what could have been discovered months earlier—that the fundamental assumptions were wrong, that users have different mental models, workflows, needs, and priorities than the team imagined.
- Temporary Enlightenment: "We should have done research first!" For a brief, shining moment, everyone agrees that understanding users before building products for them is a good idea. Executives nod sagely. Product managers take notes. The clouds part, and a ray of sunlight illuminates the path forward.
- Amnesia: Three months later, a new project begins with "We don't need research for this one..." The cycle begins anew, as if the organization has a built-in memory wipe that activates whenever lessons might actually be learned and applied.
This cycle persists because we've created corporate cultures that reward confidence over curiosity, decisiveness over deliberation, and action over understanding. The executive who declares "I know exactly what we need to build" is promoted, while the one asking "Should we check if that's what users need?" is labeled indecisive.
It's reminiscent of the old joke about the drunk looking for his keys under a streetlight. When asked if he lost them there, he says no, but the light is better. Similarly, companies keep building products based not on where user needs actually are, but where their assumptions are easiest to see.
Breaking this cycle requires a fundamental shift in how organizations think about knowledge. It requires valuing curiosity as much as conviction, questions as much as answers, and learning as much as building. It requires treating user research not as an optional step that needs ROI justification, but as an essential part of the product development process.
But until that shift happens, we researchers will continue to watch the cycle repeat, comforting ourselves with the knowledge that we'll be there to pick up the pieces when it all goes wrong—again.
The Alternative: Evidence-Based Product Development
Imagine a world where understanding users wasn't treated as an optional luxury or a mysterious black box of untraceable ROI. A world where we acknowledged that products are used by humans, and humans are complex, and perhaps talking to those humans might be a fundamental part of building things they want to use.
In this utopian vision, research isn't a separate activity with its own ROI to calculate. It's as fundamental to product development as writing code or designing interfaces. You wouldn't ask for the ROI of having developers write code that actually works—you simply understand it as a requirement for success.
This approach doesn't mean endless research cycles or analysis paralysis. It means right-sized research at the right time, focused on the questions that matter most for moving forward with confidence. It means treating user understanding as a competitive advantage rather than a cost center.
I've glimpsed this alternative reality. I once worked with a VP of Product who began every project with two questions: "What do we know about our users' needs in this area?" and "What don't we know that we should find out?" He viewed research not as a hurdle to overcome or a box to check, but as the foundation upon which good product decisions were built.
Under his leadership, the product team developed a simple framework:
- Don't know, don't build: If we don't understand the user need, we research before committing resources.
- Know, then build: If we understand the need but are unsure about the solution, we prototype and test before full development.
- Know, build, verify: Even when confident in both need and solution, we validate with users as we build.
This wasn't a radical approach—it was simply applying scientific thinking to product development. Form hypotheses, test them, learn, refine. The result? Higher adoption rates, lower support costs, and fewer failed initiatives.
The ROI wasn't calculated for each research activity because it was understood that evidence-based decision-making was simply how we worked. It would have been like asking for the ROI of using electricity in the office instead of candles.
Companies that embrace this approach tend to make fewer expensive mistakes, pivot more effectively when needed, and build stronger relationships with their users. The ROI? It's embedded in everything they do, not isolated as a separate calculation.
When organizations stop treating user research as a cost to justify and start treating it as a core capability that enables success, the entire conversation changes. The question becomes not "Can we afford to do research?" but "Can we afford to build products without understanding our users?"
Conclusion: Beyond the ROI Question
The next time someone asks you about the ROI of research, consider responding with questions of your own:
- What's the ROI of understanding the problem before trying to solve it?
- What's the ROI of not wasting resources building things no one wants?
- What's the ROI of making decisions based on evidence rather than opinions?
- What's the ROI of your company existing in six months?
The relentless pursuit of quantifying the unquantifiable reflects a fundamental misunderstanding of what research provides. It's not a direct revenue generator—it's the lens that helps focus all your revenue-generating activities on the things that actually matter to users.
Or you could just make up a number. "Our research has an ROI of 327%," you declare confidently. When asked how you calculated that, simply adjust your KPI monocle and reply, "Proprietary methodology." After all, if we're going to reduce user understanding to meaningless metrics, we might as well have fun with it.
In the meantime, those of us in the research trenches will continue our work—understanding users, identifying opportunities, preventing disasters, and occasionally banging our heads against the wall when asked to justify why understanding humans is a valuable business activity.
Because ultimately, the greatest ROI of research isn't found in any spreadsheet or dashboard. It's found in the products that fit seamlessly into users' lives, solve real problems, and keep them coming back—not because of clever marketing or growth hacks, but because we took the time to understand what they actually needed.
And if that's not worth investing in, perhaps we should all just give up and start selling blockchain-enabled virtual pet rocks. I hear there's a great ROI on those these days. Just don't ask me to show you the calculation—it's on a dashboard that only shows data on days when the market is up and Mercury is in retrograde.
Epilogue: The Research ROI Rap
(To the beat of Jay-Z's "99 Problems")
If you're having ROI problems
feel bad for you, son
I got 99 problems but research ain't one
I got execs on my back asking 'bout the numbers
Stakeholders in my ear saying "hurry up and deliver"
I got dashboards full of data but it don't explain
Why users keep bouncing from our site in pain
Product managers rush to build without knowing why
Design decisions made based on a hunch and a lie
I'm trying to get approval for some usability tests
"But what's the return?" is all that I get
If you're having ROI problems
feel bad for you, son
I got 99 problems but research ain't one
The CFO's in the room with his spreadsheet out
Demanding hard metrics for what research is about
How do you measure disasters that never occurred?
How do you quantify the bad ideas deterred?
Three months later when metrics are down
Suddenly everyone's wearing a frown
"Why aren't users engaging the way that we thought?"
Maybe it's 'cause you built what they never sought!
If you're having ROI problems
I feel bad for you, son
I got 99 problems but research ain't one
Hit me!
Next time your fancy feature fails to convert
And your NPS takes a nosedive in the dirt
Remember that budget you wouldn't approve
For understanding users before you make your move
So here's a number since that's all you need
Three hundred percent return, guaranteed!
My methodology? It's proprietary, friends
While the cost of ignorance never ends
If you're having ROI problems
I feel bad for you, son
I got 99 problems but research ain't one
🎯 Still here?
If you’ve made it this far, you probably care about users, research, and not losing your mind.
I write one longform UX essay a week — equal parts strategy, sarcasm, and survival manual.
Subscribe to get it in your inbox. No spam. No sales funnels. No inspirational LinkedIn quotes. Just real talk from the trenches.
👉 Subscribe now — before you forget or get pulled into another 87-comment Slack thread about button copy.