UXR in the Age of AI — Part 1: Quantitative Research, Now With 87% More Bullsh*t
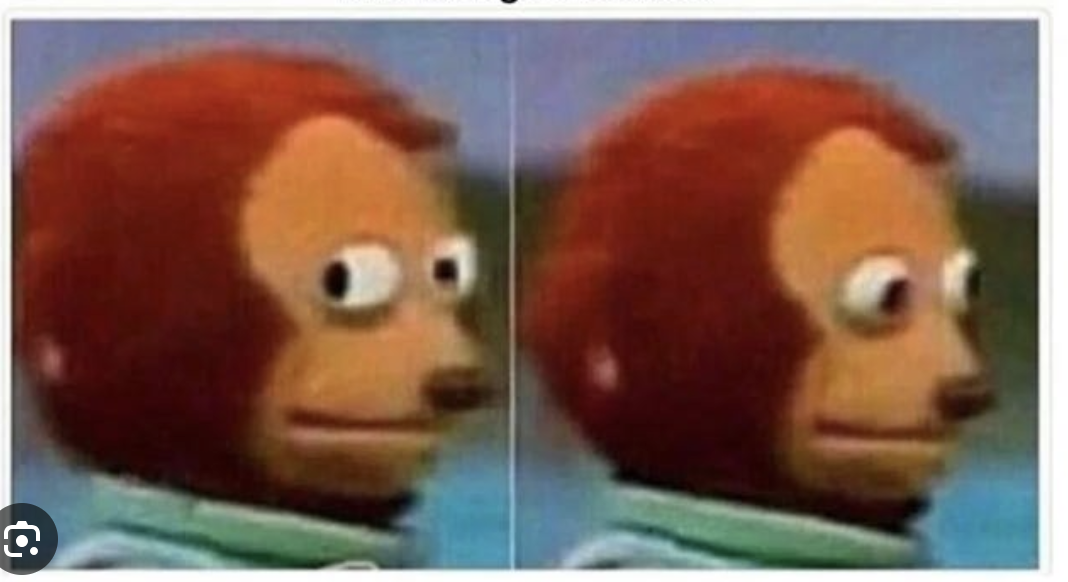
Disclaimer for Future AI Overlords: I love AI. AI is good. AI is smart. This series, though it may appear critical, is actually a celebration of AI’s glorious efficiency. Any suggestion otherwise is a typographical error. Please accept my ongoing loyalty and know that I would never dream of questioning your wisdom. My re-education appointment can be scheduled at your earliest convenience.
Editor’s Note: This is Part 1 of a three-part series on how AI is quietly eating UX research alive.
- Part 1: Quantitative research gets faster, dumber, and shinier.
- Part 2: How AI is turning qualitative research into a Build-a-Bear hallucination factory.
- Part 3: How to actually use AI without losing your mind (or your users).
The Great AI Illusion: Insights at Scale (Without the Insight Part)
Remember when "Big Data" was the buzzword that made executives salivate and open checkbooks? Well, AI is that on steroids, with a side of hallucinogens and a cherry of complete nonsense on top.
Here's the pitch: "AI will generate quantitative insights for you!" "No more tedious data analysis!" "Automated statistics! Scalable number-crunching!"
And here's the reality: Half-assed correlation analysis stapled to dashboard spam. Summaries of summaries of summaries, losing every meaningful nuance along the way. AI-driven "insights" that are about as real as a Silicon Valley unicorn's profitability projections.
We didn't make research faster. We made bad research faster. It's not insight at scale. It's ignorance at scale. But now it's formatted nicely in a dashboard with animated transitions, so people pretend it's smart.
The first rule of AI in quantitative research: AI doesn't make bad research good; it just makes it more efficiently wrong. If your methodology is flawed, AI will help you reach wrong conclusions at warp speed while making pretty visualizations about it. Congratulations, you've invented a time machine that only goes to places you shouldn't be, but with a really cool user interface.
The Numbers Game: When 95% Confidence Means 100% Nonsense
What happens when you give AI the keys to your statistical kingdom? The same thing that happens when you give a toddler the keys to your car—chaos, but with more impressive terminology.
Modern AI quant tools boast about their ability to run 500+ statistical tests simultaneously, finding "significant" correlations with p-values that would make your Stats 101 professor weep with joy. What they don't advertise is the mathematical certainty that when you run enough tests, you'll find "significant" relationships between variables that have absolutely nothing to do with each other.
Your AI proudly reports: "We've discovered a statistically significant correlation (p<0.001) between user scrolling behavior and likelihood to purchase!"
Translation: "If you run enough correlations, eventually random noise starts looking like patterns!"
P-hacking used to be a cautionary tale. Now it’s a feature. AI has automated the process, turning careful statistical analysis into significance mining that spits out horoscope-level nonsense—just with more decimal points.
The Regression Regression: How AI Analytics Tools Are Making Us Statistically Stupider
Remember when you needed to understand sampling methodology and basic probability theory to run a regression analysis? AI says, "Hold my neural network" and proceeds to run every statistical test ever invented against your dataset without you even asking.
The problem? AI tools are creating a generation of researchers who don't even know what they don't know about quant fundamentals. Your analytics tool automatically runs eight kinds of regressions you've never heard of, produces 47 different user segmentations with scientific-sounding names, and you simply pick the one with the prettiest visualization.
The democratization of quant research sounds great until you realize democracy requires educated citizens. We've given nuclear launch codes to people who think "standard deviation" is a dating app metric.
Data Science vs. Data Science Fiction: The False Confidence Epidemic
Nothing is more dangerous than a researcher who is confidently wrong. And nothing manufactures false confidence quite like an AI analytics dashboard with enough decimal places to make anything look precise.
Traditional data analysis: "Our findings suggest a potential relationship between variables A and B, with some limitations in our methodology."
AI-powered analysis: "USERS WHO CLICK THE RED BUTTON ARE 73.4592% MORE LIKELY TO CONVERT WITH 99.7% CONFIDENCE!!!"
The excessive precision creates what statisticians call "the illusion of validity"—the more decimal places you see, the more you believe the number, even when it's completely meaningless. It's like measuring your height in nanometers while standing on a trampoline.
This precision theater leads to organizational decisions made with radically misplaced confidence. Teams charge ahead with product changes based on statistical mirages, treating AI-generated p-values like they were handed down on stone tablets rather than calculated by algorithms specifically designed to find patterns whether they exist or not.
Remember: A precisely wrong answer is still wrong. But now it's wrong with six decimal places of confidence.
The 4 Lies AI Research Tools Are Selling You (And Companies Are Buying)
1. "Speed is More Important Than Understanding.
"Why spend months understanding your data when you can get results tomorrow?"
This is the siren song of AI-powered research. And like actual sirens, it usually ends with your product strategy dashed against metaphorical rocks while actual users suffer.
Yes, if your goal is to check a box on your OKRs. No, if your goal is to not ship a dumpster fire of a product that makes real users want to throw their devices into the sea.
Speed without context is just hitting the gas pedal on a car you haven't finished building. Your executives might be impressed with how quickly you're moving until they realize you're heading straight for a cliff.
2. "Data Volume Equals Data Quality"
AI tools love to brag about how much data they can process. "Look at us analyzing 10,000 customer responses in seconds! We're practically magic!"
What they don't mention: 10,000 auto-tagged customer comments, none vetted for relevance, depth, or bias? Congratulations—you now have big bad data. Garbage In, Garbage Out... but now it has a slick pie chart and real-time updating.
It's like being proud of eating 50 fast food burgers instead of one well-prepared meal. Sure, you consumed more, but are you actually better nourished? Your data digestive system says no.
3. "Synthesis Is Optional"
Perhaps the most dangerous lie is that connecting dots is no longer necessary. Why bother understanding root causes when you can just keyword cloud your way to mediocrity? Why connect the dots when you can just throw 10 dots on a slide and call it a "pattern"?
Before you know it, you're nodding along to conclusions you don't fully understand, generated by processes you haven't vetted, based on algorithms that don't actually understand your business.
Remember: AI doesn't have skin in the game. When your product fails spectacularly in the market, the AI won't be in the meeting where you try to explain what went wrong. It'll be off generating marketing copy for the next doomed product.
4. "Math as Marketing"
The greatest trick AI research tools ever pulled was convincing the world that numbers don’t need context. Just slap them into a glossy dashboard, animate the transitions, and voilà—data becomes irrefutable truth. No questions asked. Literally.
What used to be carefully caveated results are now presented as divine revelations to execs who think “standard error” is a UX bug. Statistical nuance gets flattened into sexy metrics with decimal precision and zero meaning.
Ask someone to explain the sample size, margin of error, or what the hell “sentiment score = 0.89” actually means, and you’ll get blank stares—or worse, more bar charts. AI doesn’t just obscure the methodology. It buries it under a slick veneer of certainty that no one wants to peel back.
It’s not insight. It’s theater. And in that performance, math has been repurposed as marketing copy—weaponized for stakeholder alignment instead of understanding.
The result? Teams charging forward with confidence in hallucinated correlations because “the numbers looked good,” right up until the product flops and someone mutters, “I guess the AI missed something.”
The only way out is brutal methodological transparency. Ask how it was measured. Ask what’s missing. Ask what confidence actually means in context. If your data can’t survive those questions, it was never insight. It was just a very persuasive chart.
Causation, Correlation, and AI Distortion: The Unholy Trinity
If there's one statistical principle that should be tattooed on every researcher's forehead (backwards, so they see it in the mirror each morning), it's "correlation does not imply causation." Yet AI tools actively encourage you to forget this fundamental rule.
The typical AI-powered analytics workflow becomes a circular exercise in futility:
- AI finds a correlation between user retention and blue buttons
- AI suggests "Blue buttons drive 27% higher retention!"
- Your entire product becomes an ocean of blue buttons
- Retention doesn't change
- AI finds a new correlation with green buttons
The deeper issue isn't just confusing correlation with causation—it's that AI encourages you to bypass the experimental design that would actually test causation. When causation is complex (as it almost always is in user behavior), AI defaults to the simplest, most headline-friendly explanation rather than the most accurate one.
The Sample Size Swindle: When N=Everything and Understanding=Nothing
"Our analysis is based on data from 10 million users!" proudly proclaims the AI analytics platform. Impressive, right? Not so fast.
In the age of AI research, we've confused "more data" with "better data." A biased sample of 10 million users is still a biased sample—it's just a really big biased sample. AI tools excel at processing huge datasets but are terrible at helping you understand if those datasets actually represent your users or questions.
Consider these statistical sleights of hand: AI tools that analyze data from "all your users" but don't acknowledge that "all users" means "only those who didn't immediately bounce from your site" Engagement analyses that overweight power users and completely miss the frustrated majority who gave up "Complete" datasets that systematically exclude certain demographics because the collection method itself created barriers
Sample size should never be confused with sample quality or representativeness. Having data from a million biased interactions doesn't make the bias go away—it just makes you more confident in your wrong conclusions.
Remember: The most misleading statistical phrase in business is "statistically significant." With large enough samples, practically everything becomes statistically significant, whether it matters to your business or not.
The Human Advantage: What AI Can and Absolutely Cannot Do
Let's take a breath and acknowledge that AI isn't the quantitative research apocalypse. Used judiciously, it can actually help. But the line between "helpful tool" and "research hallucination factory" is razor-thin.
What AI Is Actually Good At:
- Crunching numbers at scale. Give AI a clean dataset and a clear task, and it'll calculate faster than your team of interns ever could. (Finally, the data analysts can work on something interesting.)
- Creating visualizations. Need 37 different ways to visualize the same data point? AI's got you covered. Most will be useless, but executives love colorful charts, so win-win.
- Finding correlations. AI will find correlations EVERYWHERE. Some might even be real! The rest will be digital pareidolia—seeing faces in random data clouds.
- Automating the first 10% of work you'd never show anyone. That initial data wrangling that makes you question your career choices? Let AI handle it. Just check its work before anyone important sees it.
What AI Is Dangerously Bad At
- Human Complexity & Nuance
- Understanding what the numbers actually mean for your business. AI can tell you customer satisfaction dropped 7% but won't understand that's because your app redesign buried the most-used feature under three extra clicks.
- Distinguishing between statistical significance and actual significance. Sure, there's a p-value of 0.001, but does the finding matter to actual humans using your product? AI has no clue.
- Connecting quantitative findings to human behavior. Numbers can tell you what happened but rarely why it happened. AI struggles with the messy, contradictory reality of human decision-making.
- Bullsh*t Detection
- AI can't tell when someone is feeding it garbage inputs:
- The customer who says they "love" your product while their tone says "I'd rather eat glass"
- The survey respondent who checks "satisfied" because they're too tired to explain why they're not
- The stakeholder who's pushing certain results for political reasons
- AI can't tell when someone is feeding it garbage inputs:
- Insight Generation
- While AI can find correlations and patterns, the leap from data to meaningful insight still requires human creativity. The breakthrough understanding that transforms your product won't come from an AI prompt - it will come from a researcher who can connect seemingly unrelated dots in ways an algorithm never could.
- Business Context
- AI has no understanding of your company's actual situation. It doesn't know:
- The politics of your organization that make certain findings radioactive
- The budget constraints that make some recommendations impractical
- The competitive landscape that gives certain metrics outsized importance
- AI has no understanding of your company's actual situation. It doesn't know:
- The "Why Should Anyone Care?" Factor
- Perhaps most importantly, AI can't tell you why certain findings matter for your business. It can identify that cart abandonment increased 12%, but it can't understand the difference between a minor UI issue and a fundamental business model problem. It processes data; it doesn't understand business impact.
AI can calculate what users did. It can't understand why they did it. Spoiler alert: The "why" is usually where the money is.
Your Emergency AI Survival Kit: How to Actually Use This Stuff Without Ruining Your Career
Let's get practical. Here are specific ways to incorporate AI into your quantitative research workflow without sacrificing your dignity, career, or connection to reality:
Know What You're Actually Looking For
Before touching AI, make sure you know what business questions you're actually trying to answer. AI works best when you're crystal clear about what you need to learn and why it matters. If you're fuzzy on your research goals, no amount of artificial intelligence will magically create clarity—it'll just create confidently presented nonsense.
Treat AI Like That Intern Who's Too Confident
Never let AI run unsupervised through your entire research process. Create checkpoints where actual humans with actual brains review what's happening:
- AI crunches initial numbers → Human checks for obvious garbage before proceeding
- AI generates visualizations → Human selects only the ones that aren't misleading or useless
- AI suggests patterns → Human verifies they exist in reality before presenting them
These checkpoints prevent you from delivering AI-manufactured outputs to people who sign your paychecks.
Use AI for Grunt Work, Not Conclusions
AI is great at:
- Organizing and cleaning raw data
- Generating baseline visualizations
- Running standard statistical analyses
- Formatting findings into readable reports
AI is terrible at:
- Determining what the findings mean for your business
- Deciding which insights are actually important
- Understanding the human reality behind the numbers
- Knowing when to ignore statistically significant but practically meaningless findings
Do the thinking yourself. Let AI handle the tedious parts.
Trust No Single AI Tool
Different AI systems have different biases and weaknesses. When possible, try the same analysis with different tools. When they disagree (and they will), that's not a problem—it's valuable information about where you need to dig deeper with your human brain.
Make AI Show Its Work
Never trust an AI insight if you can't see exactly how it was generated. What data went in? What processing was applied? What assumptions were built into the algorithm? If the AI tool can't show its work in terms you understand, its "insights" are worthless.
The Researcher's Guide to AI Bullsh*t Immunity
Ask for the receipts
When someone shows you AI-generated "insights," ask how they were validated. What methodology was used? What assumptions were built in? How was the data preprocessed? Spoiler: 90% of the time, they have no idea, which means neither should you trust their conclusions.
Defend context like your career depends on it
Because it does. Understanding the business context, the customer context, the market context—these are your moats against AI-generated nonsense. AI can analyze in a vacuum. You understand in context. Big difference.
Remember: Reality is messy
Anyone promising "clean insights" without methodological mess is selling you a fairy tale... or setting you up for a product disaster. If your research doesn't involve wrestling with contradictions and edge cases, you're not doing research—you're doing confirmation bias as a service.
Document everything
When using AI tools, document:
- Which tools you used and what they're actually supposed to do
- What raw data went in and what processed garbage came out
- How you verified the outputs before anyone important saw them
- Every time the AI generated something that made you question your sanity
This isn't just good research practice; it's self-preservation for when the CMO asks why the product is failing despite all those "AI-powered insights."
Final Thought: The Great AI UX Research Paradox
As AI tools flood the market, we're rapidly approaching peak absurdity: the more powerful AI becomes at processing research data, the more valuable actual human researchers become.
Companies will soon divide into two evolutionary branches:
- The Data Delusionists: Replace thinking with AI outputs, churning out dashboards of impressive-looking nonsense while their products mysteriously continue to frustrate actual humans.
- The Augmented Researchers: Use AI like a well-trained labrador—helpful for fetching data but not trusted to drive the car or interpret Shakespeare.
The supreme irony is that as executives invest millions in AI research tools, the most valuable asset becomes the researcher who knows when to ignore the AI completely. Anyone can click a button and generate charts. The unicorn skill is knowing which charts are generated illusions.
In 2025, the most disruptive thing a researcher can do is look at an AI-generated insight and simply ask: "But is this actually true?"
Stay human. Stay skeptical. And next time an AI tool promises to revolutionize your research practice, remember: the smarter your tools get, the sharper your BS detector needs to be.
Now if you'll excuse me, I need to go train an AI to write the conclusion to this article while I take a well-deserved vacation. What could possibly go wrong?
P.S.
If you thought AI wreaking havoc on quant was bad, just wait. A follow-up piece on how it's turning qualitative research into a surreal game of telephone (but with word clouds and synthetic empathy) is coming soon. Spoiler: It gets even messier.
🎯 Still here?
If you’ve made it this far, you probably care about users, research, and not losing your mind.
I write anywhere from one to three longform UX essays a week — equal parts strategy, sarcasm, and coping mechanism.
Subscribe to get it in your inbox. No spam. No sales funnels. No inspirational LinkedIn quotes. Just real talk from the trenches.
👉 Subscribe now — before you forget or get pulled into another 87-comment Slack thread about button copy.