UXR in the Age of AI — Part 3: How AI is Collapsing the Old Field and Forcing a New One to Emerge
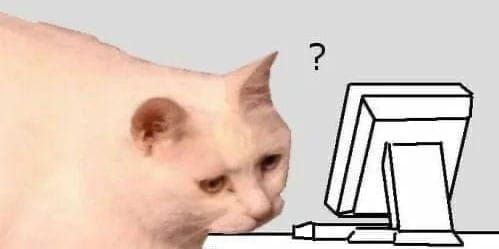
Author’s Note: This is Part 3 of my ongoing series “UXR in the Age of AI.”
If you haven’t read them yet:
- Part 1: Quantitative Research, Now With 87% More Bullsh*t looks at how AI is hollowing out shallow quant work and replacing analysis with automation theater.
- Part 2: Qualitative Research, Now With 0% Human Contact and 100% Synthetic Empathy explores the dangers of simulated empathy, fake personas, and LLM-powered research cosplay.
Both dissect how AI is transforming the work. This one? It’s about how it’s transforming the field.
The Golden Age of "Just Show Up and You're Hired"
Remember when UX Research was the Wild West of tech careers? Those days when anyone with a sociology degree and the ability to ask "How does this make you feel?" could land a six-figure job?
That openness wasn't just part of its appeal—it was the entire business model. Had a usability study on your resume? HIRED! The field needed warm bodies more than actual skills. It was still carving out legitimacy, fighting for headcount, explaining what "UXR" meant to executives who thought it was a new cryptocurrency.
Some of that work was good. Some was so bad it would make a statistics professor spontaneously combust. But if you've been on the inside, you know the dirty truth: the bar for rigor varied wildly, the title inflated quicker than a bouncy castle, and a lot of "mixed methods" was either shallow quant dressed up or qualitative research with a sprinkle of stats jargon.
"I ran a survey AND did three interviews. MIXED METHODS, BABY!"
Narrator: It was not, in fact, mixed methods.
That era is ending. Not all at once. Not with a loud layoff announcement. But with a structural shift in expectations—driven not by hiring committees with sudden standards, but by our new AI overlords who can do half our jobs while simultaneously writing poetry and planning dinner.
The Floor Just Collapsed (And Took Your Job Description With It)
AI has obliterated the barrier to entry for basic quant work faster than you can say "correlation does not imply causation" (which, let's be honest, half the field was shaky on anyway).
Suddenly, writing survey logic, cleaning up Likert data, clustering open-ends, even summarizing behavior patterns—none of that feels special anymore. It's all commoditized. Product teams are asking ChatGPT to explain churn while you're still scheduling your kickoff meeting.
So what happens next in this tragicomedy?
You get a wave of hiring managers who suddenly realize they don't need a "quant" researcher to run basic statistics. What they need is someone who can interpret, critique, and contextualize the flood of data. Someone who can see through the noise—when the dashboards lie, when the behavior misleads, when the model backfires, when the AI hallucinates that users want features that would make privacy advocates have a collective aneurysm.
And that's when the self-correction begins. Nature is healing. The UX Research ecosystem is rebalancing itself through the most efficient means possible: existential terror.
The Reckoning: When "I Know How to Use Miro" Stops Being a Career
Here's the quiet truth I've seen play out in interview rooms across startups, platforms, and consumer tech companies, where the free snacks no longer compensate for the cold sweat of imposter syndrome:
A lot of people who could pass as UXRs two years ago can't anymore.
Not because they got worse. Because the role got harder while they were busy making beautiful research repositories that nobody ever opened.
The expectations are unspoken, but very real, hanging in the air like the ghost of rejected H1 hypotheses:
- If you say "mixed methods," you better know how to debug behavioral logs, audit survey bias, and do more than just quote user sentiment while looking thoughtful. "User 3 said this feature is confusing" doesn't cut it when the AI just analyzed 50,000 support tickets in the time it took you to sip your coffee.
- If you present qualitative insights, you better tie them to risk, ROI, or roadmap tradeoffs—or they're getting filtered out faster than spam email. "Users don't like it" needs to become "This design choice will increase churn by 4% among our highest-value segments, costing approximately $3.2M in Q3."
- If you work with data scientists, you better understand how a model works, where it fails, and what the ethical cost of automation actually looks like. Otherwise, you're just the person who brings muffins to the cross-functional meeting while nodding along to terms you don't understand.
The field is bifurcating.
Not into "qual vs quant." That binary is as dead as the floppy disk. We're not in Kansas anymore, Toto.
But into those who have systems literacy—across data, design, power, and product—and those who are still waiting to be handed a script and a post-it wall while the world burns around them.
Systems literacy is knowing what happens downstream when your insight is wrong, your bias goes unchecked, or your method doesn’t scale. It’s not just knowing user needs—it’s knowing what happens when you guess.
The former will thrive. The latter are updating their resumes to emphasize transferable skills.
MM+ Is Not a Buzzword. It's a Flotation Device in the Flood.
Let's name the emerging role that might save us all from becoming AI prompt engineers: Mixed Methods+ (MM+).
The "plus" isn't flair or marketing jargon. It's the difference between career survival and joining the great resignation.
It's the ability to move across methodologies with intent and confidence. To understand which method is meaningful—not just which one is available in your company's SurveyMonkey account. To use AI tools and critique them with the ferocity of a film critic reviewing the eighth Fast & Furious movie. To hold space for user stories and convert them into quantifiable stakes that make even the most skeptical CFO pay attention.
MM+ researchers:
- don't just analyze. They interpret with the precision of someone who watches too many true crime documentaries.
- don't just collect. They triangulate data points across systems, contexts, and timeframes.
- don't just report. They frame consequences so clearly that even your CEO will understand the stakes.
And most of all—they know when a dataset, a method, or a signal is lying. They know what not to trust. That's the skill AI can't automate.
How I've Been Preparing (No Reset, Just Recalibration)
The rise of AI didn't just automate surface-level quant work. It fundamentally changed the expectations for researchers. Suddenly, it wasn't enough to know methods. You had to navigate complex systems, speak multiple organizational languages, and spot signal degradation in real time—all while the person across the table quietly wonders if GPT could do your job for cheaper.
This isn't a pivot for me. It's a continuation with sharper edges.
What that recalibration has looked like:
1. Prioritizing quant judgment over quant volume. I've stopped optimizing for scope and started sharpening interpretation. Not just "what does the data say," but "what does this trust signal mean across different user segments with varying privacy concerns?" Not every monetization insight needs a model. Some need a principled assessment of whether the data reflects actual willingness to pay or just anchoring bias from your pricing display.
2. Treating AI like a blunt instrument, not a magic wand. The tools aren't magic—they're magnifying glasses that still need a human eye to interpret what's actually important. They'll miss context, misinterpret sarcasm, and confidently present flawed conclusions. When an AI confidently declares an accessibility issue is "minor" while screen reader users can't complete critical tasks, that's when judgment matters. I incorporate these tools not because they're perfect, but because they can process scale while I focus on meaning.
3. Building a full-spectrum research tech stack. Tool fluency means independence. I use SQL not because I wanted to learn another language, but because otherwise that pattern of edge-case failures in the onboarding flow would stay hidden. I code in Python not to impress anyone, but because no one else will connect those fraud signals to legitimate user behavior if I don't. The skills aren't the point—they're just how you extract truth when regulated product spaces demand evidence nobody is collecting.
4. Becoming ruthlessly question-driven. I've shifted from method-first to question-first research. When working in AI feature design, the question isn't "do users like this interface?" but "where and how does the model output create false confidence in critical decisions?" I now spend most of my prep time pressure-testing the question itself, rather than the methodology. When stakeholders come asking for "quick user testing" on a health tech feature, my first response is always: "What patient safety risk are we trying to mitigate, and what evidence would change our approach?" This filters out the theater from the necessary.
5. Saying no to noise. If it doesn't shift a Trust & Safety roadmap, reveal unseen regulatory risk, or inform a critical monetization decision—it's gone. In spaces where errors cost more than just convenience, judgment about what matters is what makes you credible. So I cut. Relentlessly. Until only the signal remains. And AI has made this even more critical—because now we're not just drowning in data; we're drowning in auto-generated insights too. The difference is knowing which ones actually matter and which ones are just impressive-sounding fluff that will never impact the business.
This isn't a reinvention. It's a refocusing.
The work has always been complex. Now the field is catching up to what the best researchers have known all along. And I'm not here to play catch-up. I'm here to define what's next.
Final Thought: The Field is Getting Smarter. That's a Good Thing.
The shift is painful. A lot of people are getting filtered out. Some quietly, some publicly lamenting that research is "dead" when what they really mean is "the bar got higher."
But this is how disciplines evolve. This is necessary creative destruction.
UXR is growing up—not just into a technical field, but into a systems field. The researchers who survive aren't the most credentialed or the most charming. They're the ones who bring clarity to uncertainty, who can see both the edge case and the market impact.
You don’t need another certificate. You need judgment that doesn’t collapse under pressure.
If you're on this path, sharpen up. Don't chase "AI literacy" like it's the latest CrossFit trend. Build systems fluency. Don't just learn new tools. Learn new questions—the ones nobody else is asking yet.
And if you're already MM+—don't wait for the title to catch up. Do the work. Make it visible. Shape the field.
We need it.
🎯 Still clinging to post-its and “user quotes” while AI eats your job?
I write 1–3 longform essays a week—equal parts industry takedown, practical survival guide, and methodological war journal.
👉 Subscribe now if you’d rather evolve with the field than be replaced by a dashboard in a hoodie.